👨💼 การหาอนุพันธ์ย่อย (Partial differential)
มีบทบาทในงานวิศวกรรมหลายด้าน
ไม่เว้นแม้กระทั่งในวิชาคอมพิวเตอร์
ยิ่งตอนเรียนวิชาปัญญาประดิษฐ์ (AI)
สาย neural network , deep learning (กำลังดัง)
สามารถเอาไปไว้ใช้ปรับจูนพารามิเตอร์ในโมเดล
.
ในรูปที่โพสต์ต้องการหาอนพุันธ์ย่อย
หรือเรียกอีกทีก็คือให้หา gradient
หรือก็คือค่าความชัน (slope) ในวิชาเลข 📈
ถ้าพูดให้ง่ายๆ เข้าไปอีก
ในโจทย์จะให้หาความชันของ z เทียบกับ x1 และ x2
.
ซึ่งวิธีการแก้สมการไม่รู้ว่าจำกันได้ไหม
สามารถใช้พื้นฐานวิชาแคลคูลัสตอนม.ปลาย
เขาจะใช้กฏ chain rule
เป็นการหาอนุพันธ์แบบเป็นลูกโซ่
ข้อนี้ง่ายๆ แก้สมการแป็บเดียวก็เสร็จ
เอาล่ะผมคงไม่ลงคณิตศาสตร์
.
🤔 แต่อยากตั้งคำถาม
แล้วเราจะแก้โจทย์แบบนี้
ด้วยการเขียนโปรแกรมอย่างไรดี
.
😄 คำตอบ ในปัจจุบันนี้แต่ละภาษามีไลบรารี่
เอาไว้ให้แก้โจทย์ประมาณนี้อยู่แล้ว
ไม่ต้องนั่งแก้สมการทางคณิตศาสตร์ให้ปวดหัว
ขอให้เข้าใจไอเดีย ก็เขียนโปรแกรมได้ไม่ยากสบายมากๆ
.
แต่ถึงกระนั้นก็ดี
การรู้ถึงแก่นทฤษฏีในวิชาแคลคูลัส
รวมทั้งเขียนโปรแกรมแก้โจทย์ในรูปตัวอย่างได้
รับรองคุณได้ไปต่อในงาน AI ได้ไม่ยาก
.
ส่วนตัวอย่างการเขียนโปรแกรมแก้โจทย์ดังกล่าวผม
ทำตัวอย่างไว้ให้แล้วที่
https://colab.research.google.com/…/1BtVCWpwWovcVqkvEX5HaUA…
.
โค้ดสั้นๆ ไม่กี่บรรทัดเห็นจนตกใจ
เพราะตัวไลบรารี่มันหาให้เราเสร็จสรรพ
แต่ต้องเข้าใจไอเดียนะ สำคัญมากกกก
.
.
.
+++++ขอประชาสัมพันธ์ (ขายของ)
📔 หนังสือ "ปัญญาประดิษฐ์ (AI) ไม่ยาก" เข้าใจได้ด้วยเลขม. ปลาย (เนื้อหาภาษาไทย)
.
ถ้าสนใจสั่งซื้อเล่ม 1 ก็สั่งซื้อได้ที่ (เล่มอื่นๆ กำลังทยอยตามมา)
👉 https://www.mebmarket.com/web/index.php…
.
ขออภัยยังไม่มีเล่มกระดาษจำหน่าย มีแต่ ebook
.
ส่วนตัวอย่างหนังสือ ก็ดูได้ลิงค์นี้
👉 https://www.dropbox.com/s/fg8l38hc0k9b…/chapter_example.pdf…
.
✍เขียนโดย โปรแกรมเมอร์ไทย thai programmer
👨 💼 Finding sub-derivatives (Partial differential)
Role in Multiple Engineering
Not even in computer class
When I study in Artificial Intelligence (AI)
Neural network, deep learning (popular)
Can be used for tuning parameters in model
.
In the photo posted, I want to find sub-ordained.
Or call again. It's for gradient.
Or it's the steep value (slope) in math 📈
If you say it easy, go in.
In the situation, I will find z's stance against x1 and x2
.
Which is a solution to the equation. I don't know if I
Can be used in calculus course in milk. The end.
He will use the chain rule
Chain derivative findings
This one is easy. Solving the equation for a while and it will be done.
Well, I wouldn't have played math.
.
🤔 but want to question
And we will solve this situation.
With how to programming
.
😄 Answers nowadays. Each language has a library.
It's for solving this kind of problem.
No need to sit and solve a mathematical equation for headache.
If you want to understand the idea, it's not difficult. It's very easy
.
But it's good anyway.
Knowing the theory in Calculus
Including the solution programming in a sample photo.
Guarantee that you can continue in the AI job.
.
Personally, as programming solves such an issue.
Already made an example at
https://colab.research.google.com/drive/1BtVCWpwWovcVqkvEX5HaUAIyUOlekC0m#scrollTo=LWvLB33chsGI
.
A few short lines of code. I saw it until I was shocked.
Because the libraries are getting us done.
But I have to understand the idea. It's very important.
.
.
.
+++++ Public relations (selling items)
📔 The book ′′ Artificial Intelligence (AI) is not difficult It can be understood by the number. Tipping (Thai language content)
.
If you are interested in ordering book 1, you can order at (other books are gradually following).
👉 https://www.mebmarket.com/web/index.php?action=BookDetails&data=YToyOntzOjc6InVzZXJfaWQiO3M6NzoiMTcyNTQ4MyI7czo3OiJib29rX2lkIjtzOjY6IjEwODI0NiI7fQ&fbclid=IwAR11zxJea0OnJy5tbfIlSxo4UQmsemh_8TuBF0ddjJQzzliMFFoFz1AtTo4
.
Sorry, no paper books available. Only ebooks.
.
Personal like the book. You can see this link.
👉 https://www.dropbox.com/s/fg8l38hc0k9b0md/chapter_example.pdf?dl=0
.
✍ Written by Thai programmer thai coderTranslated
同時也有2部Youtube影片,追蹤數超過8萬的網紅賭Sir【杜氏數學】HermanToMath,也在其Youtube影片中提到,杜氏數學 官方網站: http://www.HermanToMath.com 賭Sir 幫你急救 DSE 數學: https://HermanToMath.skx.io ---------- ?️賭Sir是杜氏數學Herman To Math的始創人 ?全港唯一「完爆」【DSE Core+M1+M...
「mathematical model」的推薦目錄:
- 關於mathematical model 在 โปรแกรมเมอร์ไทย Thai programmer Facebook 的最讚貼文
- 關於mathematical model 在 โปรแกรมเมอร์ไทย Thai programmer Facebook 的最讚貼文
- 關於mathematical model 在 國立陽明交通大學電子工程學系及電子研究所 Facebook 的最讚貼文
- 關於mathematical model 在 賭Sir【杜氏數學】HermanToMath Youtube 的最佳解答
- 關於mathematical model 在 memehongkong Youtube 的最讚貼文
mathematical model 在 โปรแกรมเมอร์ไทย Thai programmer Facebook 的最讚貼文
👨💼 การหาอนุพันธ์ย่อย (Partial differential)
มีบทบาทในงานวิศวกรรมหลายด้าน
ไม่เว้นแม้กระทั่งในวิชาคอมพิวเตอร์
ยิ่งตอนเรียนวิชาปัญญาประดิษฐ์ (AI)
สาย neural network , deep learning (กำลังดัง)
สามารถเอาไปไว้ใช้ปรับจูนพารามิเตอร์ในโมเดล
.
ในรูปที่โพสต์ต้องการหาอนพุันธ์ย่อย
หรือเรียกอีกทีก็คือให้หา gradient
หรือก็คือค่าความชัน (slope) ในวิชาเลข 📈
ถ้าพูดให้ง่ายๆ เข้าไปอีก
ในโจทย์จะให้หาความชันของ z เทียบกับ x1 และ x2
.
ซึ่งวิธีการแก้สมการไม่รู้ว่าจำกันได้ไหม
สามารถใช้พื้นฐานวิชาแคลคูลัสตอนม.ปลาย
เขาจะใช้กฏ chain rule
เป็นการหาอนุพันธ์แบบเป็นลูกโซ่
ข้อนี้ง่ายๆ แก้สมการแป็บเดียวก็เสร็จ
เอาล่ะผมคงไม่ลงคณิตศาสตร์
.
🤔 แต่อยากตั้งคำถาม
แล้วเราจะแก้โจทย์แบบนี้
ด้วยการเขียนโปรแกรมอย่างไรดี
.
😄 คำตอบ ในปัจจุบันนี้แต่ละภาษามีไลบรารี่
เอาไว้ให้แก้โจทย์ประมาณนี้อยู่แล้ว
ไม่ต้องนั่งแก้สมการทางคณิตศาสตร์ให้ปวดหัว
ขอให้เข้าใจไอเดีย ก็เขียนโปรแกรมได้ไม่ยากสบายมากๆ
.
แต่ถึงกระนั้นก็ดี
การรู้ถึงแก่นทฤษฏีในวิชาแคลคูลัส
รวมทั้งเขียนโปรแกรมแก้โจทย์ในรูปตัวอย่างได้
รับรองคุณได้ไปต่อในงาน AI ได้ไม่ยาก
.
ส่วนตัวอย่างการเขียนโปรแกรมแก้โจทย์ดังกล่าวผม
ทำตัวอย่างไว้ให้แล้วที่
https://colab.research.google.com/…/1BtVCWpwWovcVqkvEX5HaUA…
.
โค้ดสั้นๆ ไม่กี่บรรทัดเห็นจนตกใจ
เพราะตัวไลบรารี่มันหาให้เราเสร็จสรรพ
แต่ต้องเข้าใจไอเดียนะ สำคัญมากกกก
.
.
.
+++++ขอประชาสัมพันธ์ (ขายของ)
📔 หนังสือ "ปัญญาประดิษฐ์ (AI) ไม่ยาก" เข้าใจได้ด้วยเลขม. ปลาย (เนื้อหาภาษาไทย)
.
ถ้าสนใจสั่งซื้อเล่ม 1 ก็สั่งซื้อได้ที่ (เล่มอื่นๆ กำลังทยอยตามมา)
👉 https://www.mebmarket.com/web/index.php…
.
ขออภัยยังไม่มีเล่มกระดาษจำหน่าย มีแต่ ebook
.
ส่วนตัวอย่างหนังสือ ก็ดูได้ลิงค์นี้
👉 https://www.dropbox.com/s/fg8l38hc0k9b…/chapter_example.pdf…
.
✍เขียนโดย โปรแกรมเมอร์ไทย thai programmer
👨 💼 Finding sub-derivatives (Partial differential)
Role in Multiple Engineering
Not even in computer class
When I study in Artificial Intelligence (AI)
Neural network, deep learning (popular)
Can be used for tuning parameters in model
.
In the photo posted, I want to find sub-ordained.
Or call again. It's for gradient.
Or it's the steep value (slope) in math 📈
If you say it easy, go in.
In the situation, I will find z's stance against x1 and x2
.
Which is a solution to the equation. I don't know if I
Can be used in calculus course in milk. The end.
He will use the chain rule
Chain derivative findings
This one is easy. Solving the equation for a while and it will be done.
Well, I wouldn't have played math.
.
🤔 but want to question
And we will solve this situation.
With how to programming
.
😄 Answers nowadays. Each language has a library.
It's for solving this kind of problem.
No need to sit and solve a mathematical equation for headache.
If you want to understand the idea, it's not difficult. It's very easy
.
But it's good anyway.
Knowing the theory in Calculus
Including the solution programming in a sample photo.
Guarantee that you can continue in the AI job.
.
Personally, as programming solves such an issue.
Already made an example at
https://colab.research.google.com/drive/1BtVCWpwWovcVqkvEX5HaUAIyUOlekC0m#scrollTo=LWvLB33chsGI
.
A few short lines of code. I saw it until I was shocked.
Because the libraries are getting us done.
But I have to understand the idea. It's very important.
.
.
.
+++++ Public relations (selling items)
📔 The book ′′ Artificial Intelligence (AI) is not difficult It can be understood by the number. Tipping (Thai language content)
.
If you are interested in ordering book 1, you can order at (other books are gradually following).
👉 https://www.mebmarket.com/web/index.php?action=BookDetails&data=YToyOntzOjc6InVzZXJfaWQiO3M6NzoiMTcyNTQ4MyI7czo3OiJib29rX2lkIjtzOjY6IjEwODI0NiI7fQ&fbclid=IwAR11zxJea0OnJy5tbfIlSxo4UQmsemh_8TuBF0ddjJQzzliMFFoFz1AtTo4
.
Sorry, no paper books available. Only ebooks.
.
Personal like the book. You can see this link.
👉 https://www.dropbox.com/s/fg8l38hc0k9b0md/chapter_example.pdf?dl=0
.
✍ Written by Thai programmer thai coderTranslated
mathematical model 在 國立陽明交通大學電子工程學系及電子研究所 Facebook 的最讚貼文
【演講】2019/11/19 (二) @工四816 (智易空間),邀請到Prof. Geoffrey Li(Georgia Tech, USA)與Prof. Li-Chun Wang(NCTU, Taiwan) 演講「Deep Learning based Wireless Resource Allocation/Deep Learning in Physical Layer Communications/Machine Learning Interference Management」
IBM中心特別邀請到Prof. Geoffrey Li(Georgia Tech, USA)與Prof. Li-Chun Wang(NCTU, Taiwan)前來為我們演講,歡迎有興趣的老師與同學報名參加!
演講標題:Deep Learning based Wireless Resource Allocation/Deep Learning in Physical Layer Communications/Machine Learning Interference Management
演 講 者:Prof. Geoffrey Li與Prof. Li-Chun Wang
時 間:2019/11/19(二) 9:00 ~ 12:00
地 點:交大工程四館816 (智易空間)
活動報名網址:https://forms.gle/vUr3kYBDB2vvKtca6
報名方式:
費用:(費用含講義、午餐及茶水)
1.費用:(1) 校內學生免費,校外學生300元/人 (2) 業界人士與老師1500/人
2.人數:60人,依完成報名順序錄取(完成繳費者始完成報名程序)
※報名及繳費方式:
1.報名:請至報名網址填寫資料
2.繳費:
(1)親至交大工程四館813室完成繳費(前來繳費者請先致電)
(2)匯款資訊如下:
戶名: 曾紫玲(國泰世華銀行 竹科分行013)
帳號: 075506235774 (國泰世華銀行 竹科分行013)
匯款後請提供姓名、匯款時間以及匯款帳號後五碼以便對帳
※將於上課日發放課程繳費領據
聯絡方式:曾紫玲 Tel:03-5712121分機54599 Email:tzuling@nctu.edu.tw
Abstract:
1.Deep Learning based Wireless Resource Allocation
【Abstract】
Judicious resource allocation is critical to mitigating interference, improving network efficiency, and ultimately optimizing wireless network performance. The traditional wisdom is to explicitly formulate resource allocation as an optimization problem and then exploit mathematical programming to solve it to a certain level of optimality. However, as wireless networks become increasingly diverse and complex, such as high-mobility vehicular networks, the current design methodologies face significant challenges and thus call for rethinking of the traditional design philosophy. Meanwhile, deep learning represents a promising alternative due to its remarkable power to leverage data for problem solving. In this talk, I will present our research progress in deep learning based wireless resource allocation. Deep learning can help solve optimization problems for resource allocation or can be directly used for resource allocation. We will first present our research results in using deep learning to solve linear sum assignment problems (LSAP) and reduce the complexity of mixed integer non-linear programming (MINLP), and introduce graph embedding for wireless link scheduling. We will then discuss how to use deep reinforcement learning directly for wireless resource allocation with application in vehicular networks.
2.Deep Learning in Physical Layer Communications
【Abstract】
It has been demonstrated recently that deep learning (DL) has great potentials to break the bottleneck of the conventional communication systems. In this talk, we present our recent work in DL in physical layer communications. DL can improve the performance of each individual (traditional) block in the conventional communication systems or jointly optimize the whole transmitter or receiver. Therefore, we can categorize the applications of DL in physical layer communications into with and without block processing structures. For DL based communication systems with block structures, we present joint channel estimation and signal detection based on a fully connected deep neural network, model-drive DL for signal detection, and some experimental results. For those without block structures, we provide our recent endeavors in developing end-to-end learning communication systems with the help of deep reinforcement learning (DRL) and generative adversarial net (GAN). At the end of the talk, we provide some potential research topics in the area.
3.Machine Learning Interference Management
【Abstract】
In this talk, we discuss how machine learning algorithms can address the performance issues of high-capacity ultra-dense small cells in an environment with dynamical traffic patterns and time-varying channel conditions. We introduce a bi adaptive self-organizing network (Bi-SON) to exploit the power of data-driven resource management in ultra-dense small cells (UDSC). On top of the Bi-SON framework, we further develop an affinity propagation unsupervised learning algorithm to improve energy efficiency and reduce interference of the operator deployed and the plug-and-play small cells, respectively. Finally, we discuss the opportunities and challenges of reinforcement learning and deep reinforcement learning (DRL) in more decentralized, ad-hoc, and autonomous modern networks, such as Internet of things (IoT), vehicle -to-vehicle networks, and unmanned aerial vehicle (UAV) networks.
Bio:
Dr. Geoffrey Li is a Professor with the School of Electrical and Computer Engineering at Georgia Institute of Technology. He was with AT&T Labs – Research for five years before joining Georgia Tech in 2000. His general research interests include statistical signal processing and machine learning for wireless communications. In these areas, he has published around 500 referred journal and conference papers in addition to over 40 granted patents. His publications have cited by 37,000 times and he has been listed as the World’s Most Influential Scientific Mind, also known as a Highly-Cited Researcher, by Thomson Reuters almost every year since 2001. He has been an IEEE Fellow since 2006. He received 2010 IEEE ComSoc Stephen O. Rice Prize Paper Award, 2013 IEEE VTS James Evans Avant Garde Award, 2014 IEEE VTS Jack Neubauer Memorial Award, 2017 IEEE ComSoc Award for Advances in Communication, and 2017 IEEE SPS Donald G. Fink Overview Paper Award. He also won the 2015 Distinguished Faculty Achievement Award from the School of Electrical and Computer Engineering, Georgia Tech.
Li-Chun Wang (M'96 -- SM'06 -- F'11) received Ph. D. degree from the Georgia Institute of Technology, Atlanta, in 1996. From 1996 to 2000, he was with AT&T Laboratories, where he was a Senior Technical Staff Member in the Wireless Communications Research Department. Currently, he is the Chair Professor of the Department of Electrical and Computer Engineering and the Director of Big Data Research Center of of National Chiao Tung University in Taiwan. Dr. Wang was elected to the IEEE Fellow in 2011 for his contributions to cellular architectures and radio resource management in wireless networks. He was the co-recipients of IEEE Communications Society Asia-Pacific Board Best Award (2015), Y. Z. Hsu Scientific Paper Award (2013), and IEEE Jack Neubauer Best Paper Award (1997). He won the Distinguished Research Award of Ministry of Science and Technology in Taiwan twice (2012 and 2016). He is currently the associate editor of IEEE Transaction on Cognitive Communications and Networks. His current research interests are in the areas of software-defined mobile networks, heterogeneous networks, and data-driven intelligent wireless communications. He holds 23 US patents, and have published over 300 journal and conference papers, and co-edited a book, “Key Technologies for 5G Wireless Systems,” (Cambridge University Press 2017).
mathematical model 在 賭Sir【杜氏數學】HermanToMath Youtube 的最佳解答
杜氏數學 官方網站: http://www.HermanToMath.com
賭Sir 幫你急救 DSE 數學: https://HermanToMath.skx.io
----------
?️賭Sir是杜氏數學Herman To Math的始創人
?全港唯一「完爆」【DSE Core+M1+M2】、【IAL 12科Maths】、【AL Pure+Applied】、【CE Maths+A.Maths】的數學導師
?全港第一最多訂閱粉絲的數學教育YouTuber
?YouTube觀看次數超越700萬、訂閱粉絲超過50000人
?著作:《YouTuber新手到網紅》、《5**數學男人嫁得過》、《碌葛男人嫁得過》、《賭波男人嫁得過》(獲Google嚴選2018年度50大最佳書籍)
----------
賭Sir收集著數派街坊:
❤️YouTuber Go網絡課程 全港最平+獨家 報讀優惠:
?報讀初班 $600 (原價$800):https://www.youtubergo.com/payment/b-hermantomath-0600.html
?報讀初班+中班 $1500 (原價$1800):https://www.youtubergo.com/payment/bm-hermantomath-1500.html
官方網頁:https://www.youtubergo.com/
❤️無限操數王(epractice) 全港最平+獨家 優惠(可同時使用):
?50%OFF 半價優惠碼:MC83-AI93-NFW0-331E
?25%OFF 額外邀請碼:J7N9-RDRP-NFAH-OH13
官方網頁:https://www.dsemth.com/
❤️Tidebit全港最穩妥的比特幣(Bitcoin)交易所:http://bit.ly/2LIWA4J
❤️Uber免費送你$25優惠:https://www.uber.com/invite/2utyzr
----------
杜氏數學 國際官方網站 http://www.hermantomath.com
----------
Title:
賭場VS賭波VS賭馬,如何預測賽果?
----------
Subtitle:
天有不測之風雲,何以天文台能夠預測天氣?
----------
Script:
賭場、賭波和賭馬,形式上非常不同:
賭局 賠率 機率
賭場遊戲 己知 己知
足球博彩 己知 未知
賽馬博彩 未知 未知
由於形式不同,戰術亦截然不同。但戰術不同,目標卻始終如一:「正EV」。只要EV是正數,賭博便佔優。重溫一次EV的計算方法:
EV = 淨贏注 × 贏錢機率 - 淨輸注 × 輸錢機率
換言之,賭場遊戲的賠率固定、機率固定,因此EV都是固定,而且一般來說都是固定的負數(因為對賭場來說便是正數)。對賭客來說,除非遇上賭場犯錯,例如推出新遊戲,規則上容許賭客獲得正EV#,否則於賭場遊戲長賭必敗無疑。
#《爽爆:全職賭徒鑽賭場漏洞 月贏80萬 》
http://hk.apple.nextmedia.com/news/art/20121017/18042618
至於足球博彩,雖然是固定賠率制,但由於足球比賽變化莫測,不似賭場遊戲純粹訴諸物理力學,因此機率是未知之數,自然EV也是未知之數。只要有一定方法,便有可能取得正EV。
或許你會問:既然足球比賽變化莫測,一個不慎擺烏龍、一個不智領紅牌、一個球證誤判越位入球等,都會影響賽果,試問又怎能夠計算呢?
這個問題就等如天有不測之風雲,天文台為何能夠預測天氣呢?當然間中亦有錯判,但雖不中亦不遠矣,這就是數學的力量。其實博彩公司訂立賠率的時候,都會先以數學計算賽果機率,然後輕微調低作抽水。由此可見,只要比博彩公司計算機率計算得更準確,便能夠於賭局中佔有上風。
舉個例,朋友和你在街頭足球場看見兩隊業餘球隊比賽,朋友見一隊年輕力壯,另一隊白髮蒼蒼,於是開盤:「年輕隊1賠0.8、和局1賠2.1、元老隊1賠3.1」,這個時候,你發現元老隊球員原來是前甲組職業球員,年輕隊則是自己兒子的球隊,而你知道自己的兒子和他的朋友是乒乓球隊友,根本不懂得踢足球,因此,你預算元老隊的勝率遠超年輕隊。明顯地,這個賭局是你佔了上風。
換言之,這是一場資訊(Information)戰,擁有更多資訊的佔優。為什麼?因為資訊較多的一方,更能較準確地計算賽局機率(這也是馬評家晨早起床看晨操的目的,獲取一般馬迷不知道的資訊)。於上述例子,雖然不涉及數學運算,但仍算是一種粗略估算。當然,面對博彩公司,粗略估算是不足夠的,你需要比博彩公司更精確的機率計算,而方法就是:建立一個數學模型(Mathematical Model)。
提供重要資訊
計算預測結果
你先從現實世界搜集重要資訊,例如對賽兩隊的近績、對賽往績、預計陣容等,而對賽果影響力較小的,可選擇性地抽取,例如天氣溫度、領隊教練、花邊新聞等。然後,將這些資訊輸入到電腦程式,並由電腦進行運算,得出答案後,把電腦程式輸出的賽果,視之為對現實世界的預測賽果。程序大致如此,天文台預測天氣也是透過數學建模(Mathematical Modeling),量化重要的氣候現象,來預測未來天氣。
然而,電腦程式是如何使用現實資訊的呢?首先預設一些公式,然後匯入大量球賽歷史資訊,例如上述的近績、對賽往績、甚至天氣溫度等,從而利用公式計算預測賽果,將它與真實賽果比較,便可得知每一條公式的預測準繩度,繼而從中選出預測力最高的公式,加以使用,計算EV。
最常見的疑問是:「公式的準繩度源於球賽歷史資訊,包括真實賽果,準繩度自然必被高估,試問對比真實賽果又有什麼意思?」
這個問題可以利用一個名叫回溯測試(Backtesting)的小聰明手法,匯入資訊時,只匯入一部份,留下剩餘的部份歷史賽事當作未來賽事,執行公式模擬投注。
舉例說,你找了1000場相關賽事,你可匯入首900場,來挑選公式,然後用尾100場作模擬投注,計算出使用公式的EV。
賽馬博彩也是透過數學建模,你除了需要計算機率之外,你也要模擬最後賠率。因為賽馬博彩是實行彩池制(Pari Mutuel,又稱同注分彩法),賠率會因應投注額的分佈而時刻調整。假設你投注的時候,一號馬是1賠10,臨開閘的時候可以變了1賠3,到最後派彩可以變了1賠6,而你最後獲得的賠率,就是根據最後派彩,而不是你投注的時候。
由此可見,如使用數學建模,賭馬比賭波容易獲得正EV。主要原因如下:
賽馬是賭客與賭客之間的對賭。實施彩池制,博彩公司抽取投注額的手續費獲利,無論賽果如何,博彩公司已經賺了,派彩只是用輸家的注碼賠給贏家。只要有大量非理性的賭客,賭局佔優的機率便會較高,就好像到麻雀館打麻雀,遇著三位菜鳥,贏面自然較高。
相反,足球博彩是固定賠率制,是莊家和賭客直接對賭,莊家自然費盡工夫調整盤口,為公司獲得正EV,博彩公司正EV,即是賭客負EV。要從足球博彩中使用數學模型取勝,就得比博彩公司計算得更精確才有機會成功。
實際操作上,數學模型的構造當然比以上描述複雜得多,例如考慮的因素、各個因素的比重、賽事的數量,甚至注碼大小等,都絕不簡單。然而,原理大致上就是如此。
這一堂不教任何數學建模的方法,因為所需要的數學水平起碼要有大學程度,如想擊敗賭場,開始學習數學吧,有心不怕遲,只要沒有了考試的壓力,學習數學其實很愉快,也很輕鬆,或許最後你做不了賭神,卻成了數學家呢!
就算不打算學習數學,也希望你明白背後的原理,不致於大庭廣眾之下獻醜,不會再說由於隨機因此無法預測,而別人提起數學模型的時候,你起碼聽得明白。
天氣預測的科學發展已成熟多年,人類掌控隨機事件的能力已遠超一般人所想。天文台雖然無法完美預測每一秒的天氣變化,但大概準確,已造福人群;同樣地,賭局預測,雖然不會場場中,但只要大概準確,使贏的多過輸的,已足夠使賭客獲利。數學並非萬能,但只要適當地使用,絕對是強大的武器。
Summary
賭場遊戲的賠率和機率都是固定。
足球博彩實行固定賠率制(Fixed-odds betting),賠率固定,但機率不知。
賽馬博彩實行彩池制,賠率不定,機率亦不知。
賽果預測的原理,與天氣預測的原理大致相同。
將現實世界重要資訊,匯入數學模型計算,用結果預測現實世界賽果。
把部份歷史賽事當作未來賽事,用以驗證數學程式的準繩度。
天氣預測無須分秒不差,賭局預測亦無須場場中,只要正EV就可以。
Terminology
資訊(Information)
數學模型(Mathematical Model)
數學建模(Mathematical Modeling)
回溯測試(Backtesting)
彩池制(Pari Mutuel)
固定賠率制(Fixed-odds betting)
-----------
杜氏數學 Herman To Math 考試戰績:
A ── 會考 Math 數學
A ── 會考 Additional Math 附加數學
A ── 高考 Pure Math 純粹數學
A ── 高考 Applied Math 應用數學
5** ── DSE Math 數學
5** ── DSE M1 數學延伸部分(一)
5** ── DSE M2 數學延伸部分(二)
A ── IAL Core Math 1 2
A ── IAL Core Math 3 4
A ── IAL Further Pure Math 1
A ── IAL Mechanics 2
A ── IAL Mechanics 3
A ── IAL Statistics 1
A ── IAL Statistics 2
----------
精選系列節錄:
《賭Sir數學戒賭》糸列
https://www.youtube.com/watch?v=dhL-dRcIN5I&index=1&list=PL_CM4U5au2k1cfK2zSph8XOLqIjOPQmvo
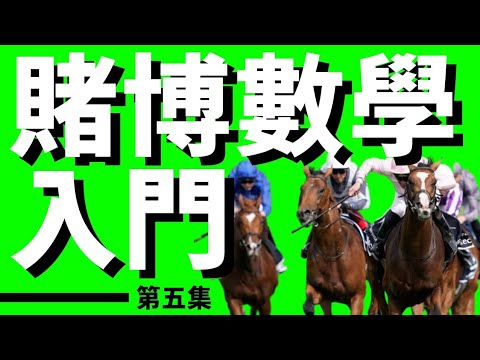
mathematical model 在 memehongkong Youtube 的最讚貼文
我想講講諾貝爾物理學獎,因為希格斯獲獎,這是一件很重大的事。首先,希格斯發明希格斯粒子。我們知道世界是充滿力量,所謂的四大力量,分別是重力、電磁力、強作用力和弱作用力。現在有個standard model把三種力量合而為一。強作用力、弱作用力和電磁力是一種力量來的。但沒有人明白重力是甚麼。究竟重力是甚麼東西。所謂的大統一論,意圖把重力收歸入去,但在量子力學的層次中,這仍是未能成功的。那重力是甚麼?這又牽涉到質量究竟是甚麼?質量不是一種力。你有多少mass,但mass不是一種力。希格斯用他的mathematical formula發明了原來所謂質量。這個世界是充滿了一種你看不見的粒子,叫做希格斯粒子。所有所謂的質量,好像阿mark的重量,質量是不變的,但在地球上的是重量,因為希格斯粒子充滿在宇宙之間,所有粒子都會和他產生一種interative的力量,於是你便感覺到它有質量。一個簡單的例子,你在空氣中行走,你會快很多,但是你在水中會慢很多,因為水的質量高一點。而你在膠水中,你甚至會動不了,於是你覺得自己重了很多。這個是假象。其實是因為希格斯粒子和你身上產生一種互動的力量,所以產生了質量。
這又要講回世界上的物理學家可以分為三種。一種叫理論物理學家。一種叫做實驗物理學家。理論物理學家發明東西出來,基本上很多是靠數學而來的,是不可以獲得諾貝爾獎,除非有實驗證明了,或者實驗出現突破,證明到以前不能證明的事,譬如話找到宇宙微波恒數,那你就可以得獎。所以希格斯為何現在才得獎,因為過去兩年瑞士的強子撞擊機找到希格斯粒子。為何霍金到現在也不能得獎,因為他的黑洞消散理論沒有經過實驗的驗證。沒有實驗驗證,那不過是一個數學model。所以為何Witten這個世界上最聰明的人也得不到獎,他的超級弦理,只是一套formula,沒有任何實驗證明到他是存在的。好像愛因斯坦,終於在那次日蝕證明了光是會彎曲,然後他才得到全世界的接受。第三種是實用物理學家,他們把這些理論套用到實用上。這些更難得獎,非常難得獎。這只是得一個我們認識的人,就是高錕。他把物理用在光纖上,對我們的生活影響太大,所以他很例外地得到物理學獎。
謎米香港 www.memehk.com
Facebook: www.facebook.com/memehkdotcom

mathematical model 在 What is Mathematical Model | IGI Global 的相關結果
What is Mathematical Model? Definition of Mathematical Model: Description of a system using mathematical concepts and language. ... <看更多>
mathematical model 在 An Introduction to Mathematical Modelling 的相關結果
1.1 What is mathematical modelling? Models describe our beliefs about how the world functions. In mathematical modelling, we translate those beliefs into the ... ... <看更多>
mathematical model 在 Mathematical model - Wikipedia 的相關結果
A mathematical model is a description of a system using mathematical concepts and language. The process of developing a mathematical model is termed ... ... <看更多>